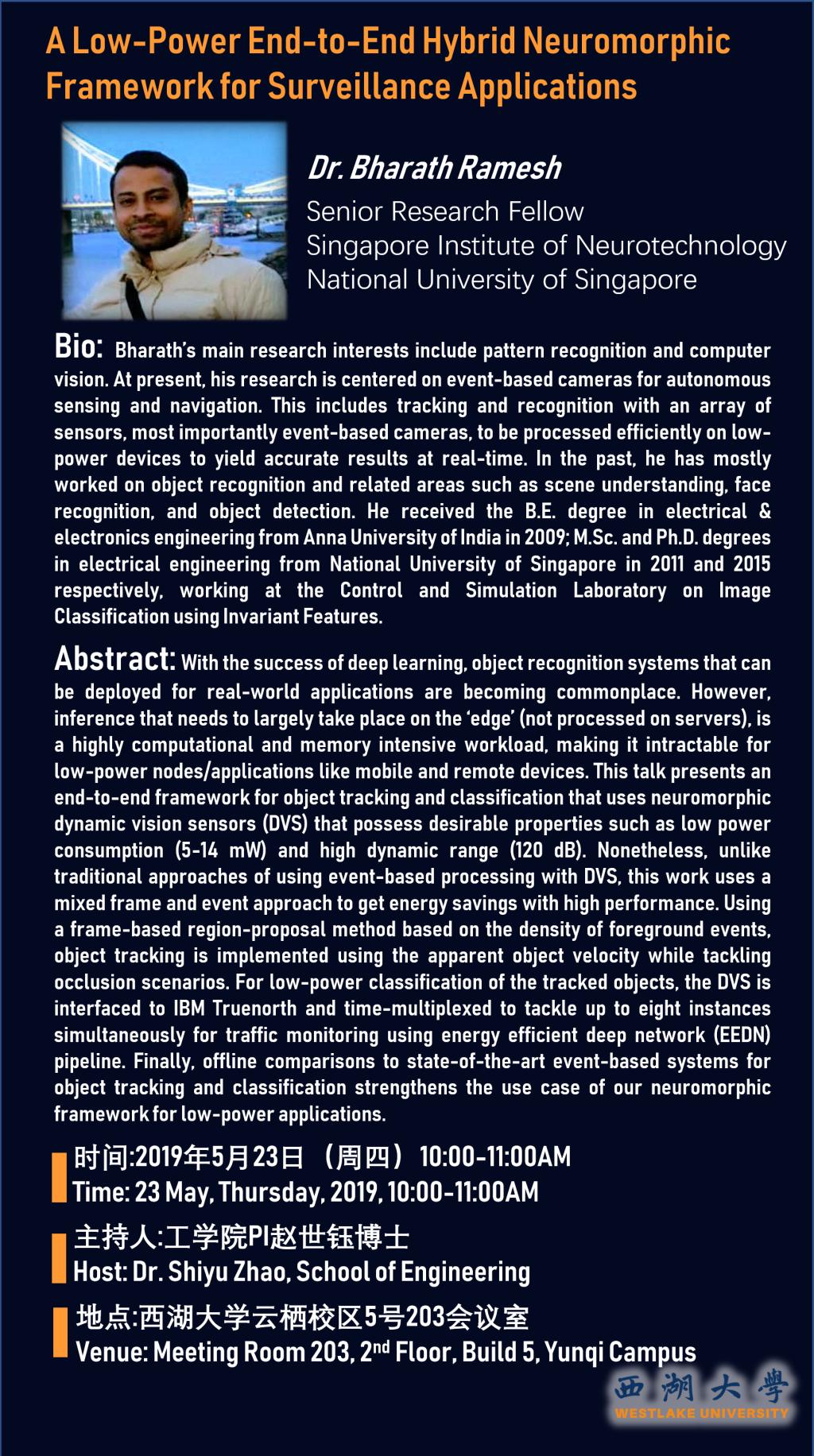
Bio:
Bharath’s main research interests include pattern recognition and computer vision. At present, his research is centered on event-based cameras for autonomous sensing and navigation. This includes tracking and recognition with an array of sensors, most importantly event-based cameras, to be processed efficiently on low-power devices to yield accurate results at real-time. In the past, he has mostly worked on object recognition and related areas such as scene understanding, face recognition, and object detection. He received the B.E. degree in electrical & electronics engineering from Anna University of India in 2009; M.Sc. and Ph.D. degrees in electrical engineering from National University of Singapore in 2011 and 2015 respectively, working at the Control and Simulation Laboratory on Image Classification using Invariant Features.
Abstract:
With the success of deep learning, object recognition systems that can be deployed for real-world applications are becoming commonplace. However, inference that needs to largely take place on the ‘edge’ (not processed on servers), is a highly computational and memory intensive workload, making it intractable for low-power nodes/applications like mobile and remote devices. This talk presents an end-to-end framework for object tracking and classification that uses neuromorphic dynamic vision sensors (DVS) that possess desirable properties such as low power consumption (5-14 mW) and high dynamic range (120 dB). Nonetheless, unlike traditional approaches of using event-based processing with DVS, this work uses a mixed frame and event approach to get energy savings with high performance. Using a frame-based region-proposal method based on the density of foreground events, object tracking is implemented using the apparent object velocity while tackling occlusion scenarios. For low-power classification of the tracked objects, the DVS is interfaced to IBM Truenorth and time-multiplexed to tackle up to eight instances simultaneously for traffic monitoring using energy efficient deep network (EEDN) pipeline. Finally, offline comparisons to state-of-the-art event-based systems for object tracking and classification strengthens the use case of our neuromorphic framework for low-power applications.
时间:2019年5月23日(周四)10:00-11:00AM
Time: 23 May, Thursday, 2019, 10:00-11:00AM
主持人:工学院PI赵世钰博士
Host: Dr. Shiyu Zhao, School of Engineering
地点:西湖大学云栖校区5号203会议室
Venue: Meeting Room 203, 2nd Floor, Build 5, Yunqi Campus