5月13日,英国拉夫堡大学陈文华教授(IEEE Fellow)受邀来访西湖大学智能无人系统实验室。陈教授在无人机系统和智能车辆技术开发方面拥有20多年的科研经验,涵盖了自动驾驶、情境感知、决策控制、精准农业和环境监测的遥感技术,特别是在控制、信号处理和人工智能方面具有丰富的应用经验。
图1 讲座开场介绍环节 图2 讲座现场的师生
在今天的讲座中,陈教授详细阐述了他们的最新研究成果——一种基于利用和探测的最优双重控制算法。该算法既能充分利用已经测量到的信息,还能主动探索未知区域。因此,该算法的优势在于它不仅保持了传统控制理论的稳定性,还融合了强化学习算法的适应性,有效解决了未知且复杂场景中的自适应控制难题。该算法集成了感知、估计和控制等多个领域,构建了一套完整的全自动机器人系统,可以直接应用到现实场景中,具有很高的实际应用价值。陈教授的研究成果与我们实验室的研究方向高度契合,为我们带来了诸多启发,尤其是在无人机跟踪场景中的未知目标运动估计问题。
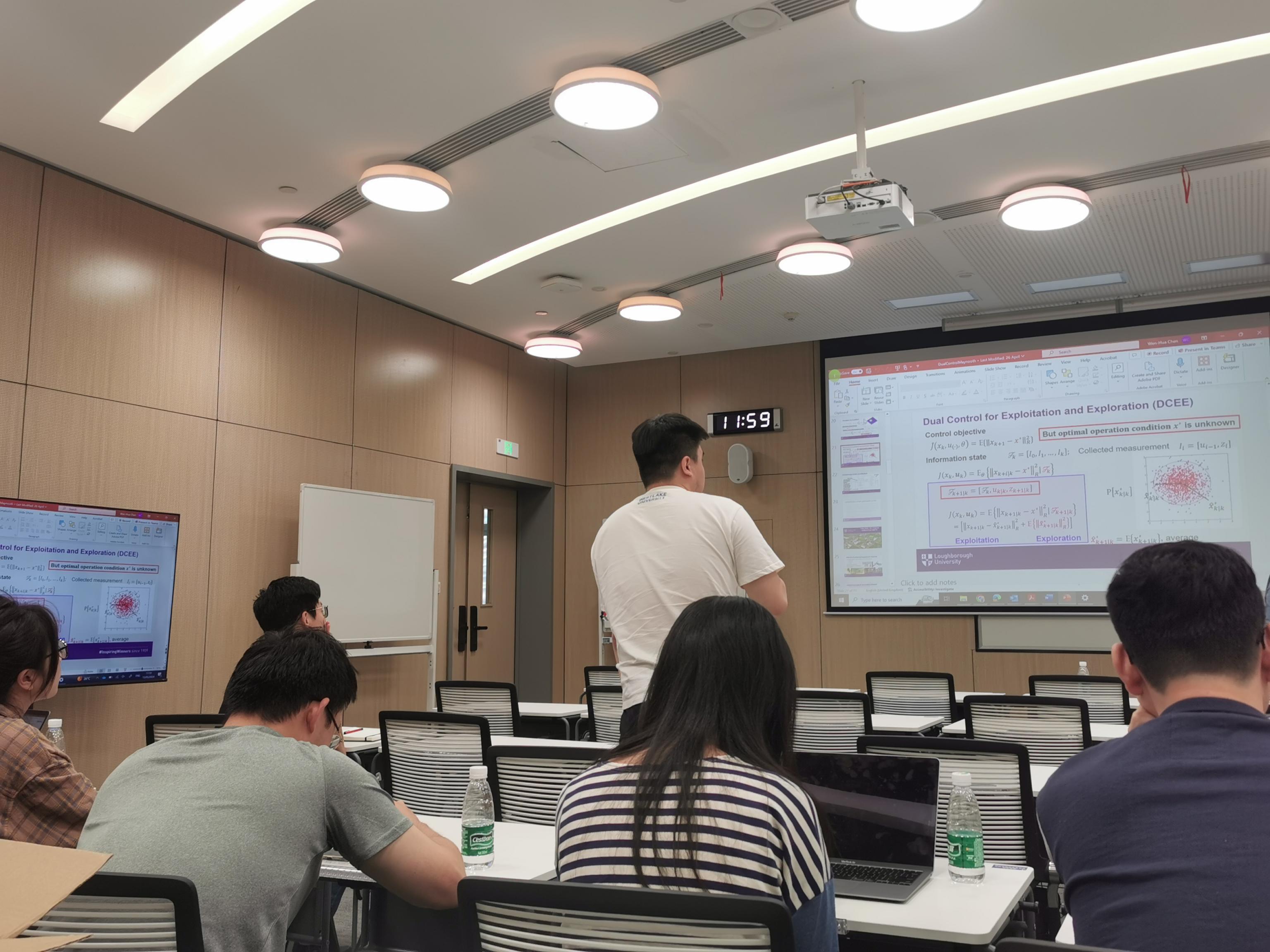
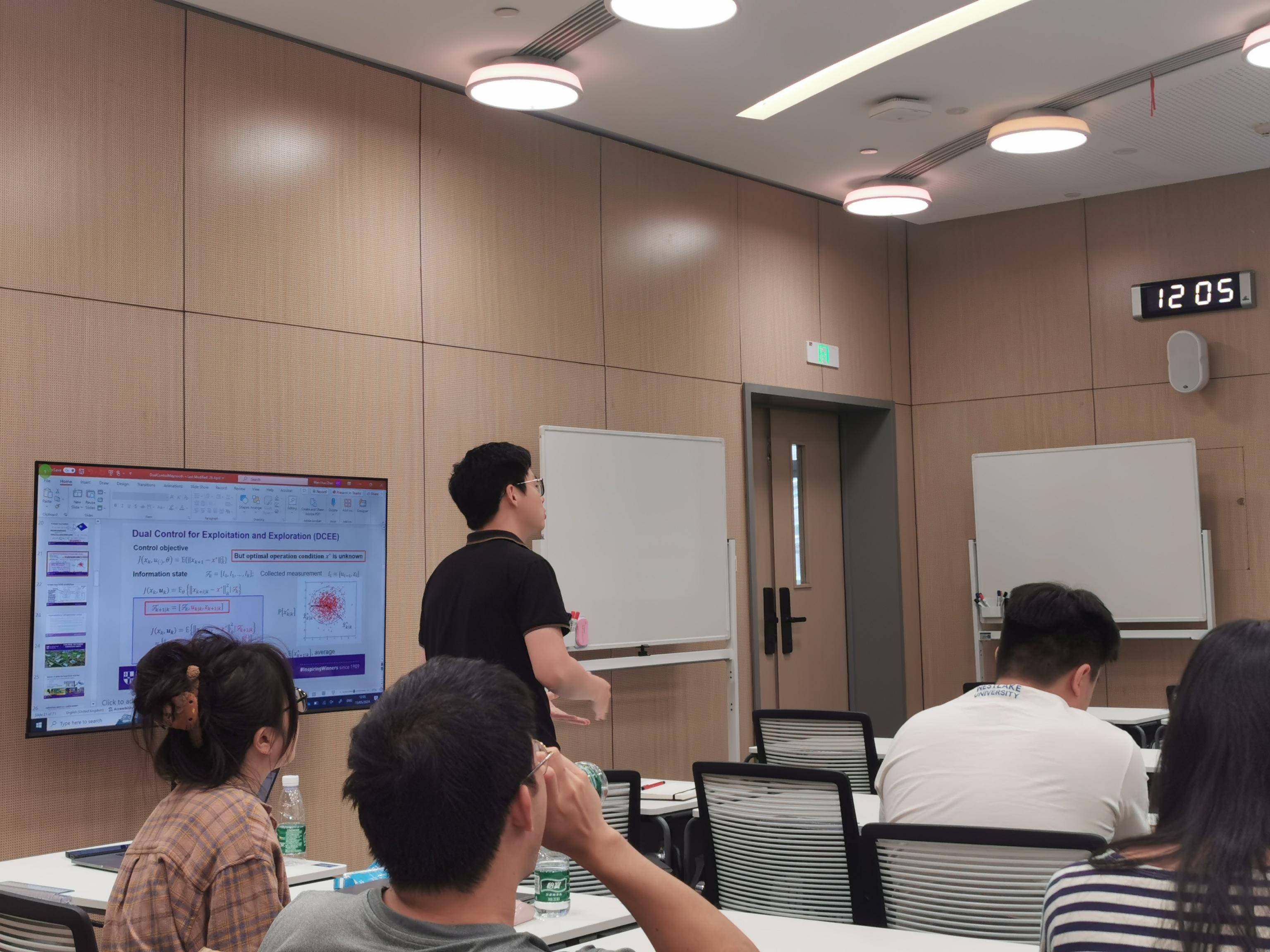
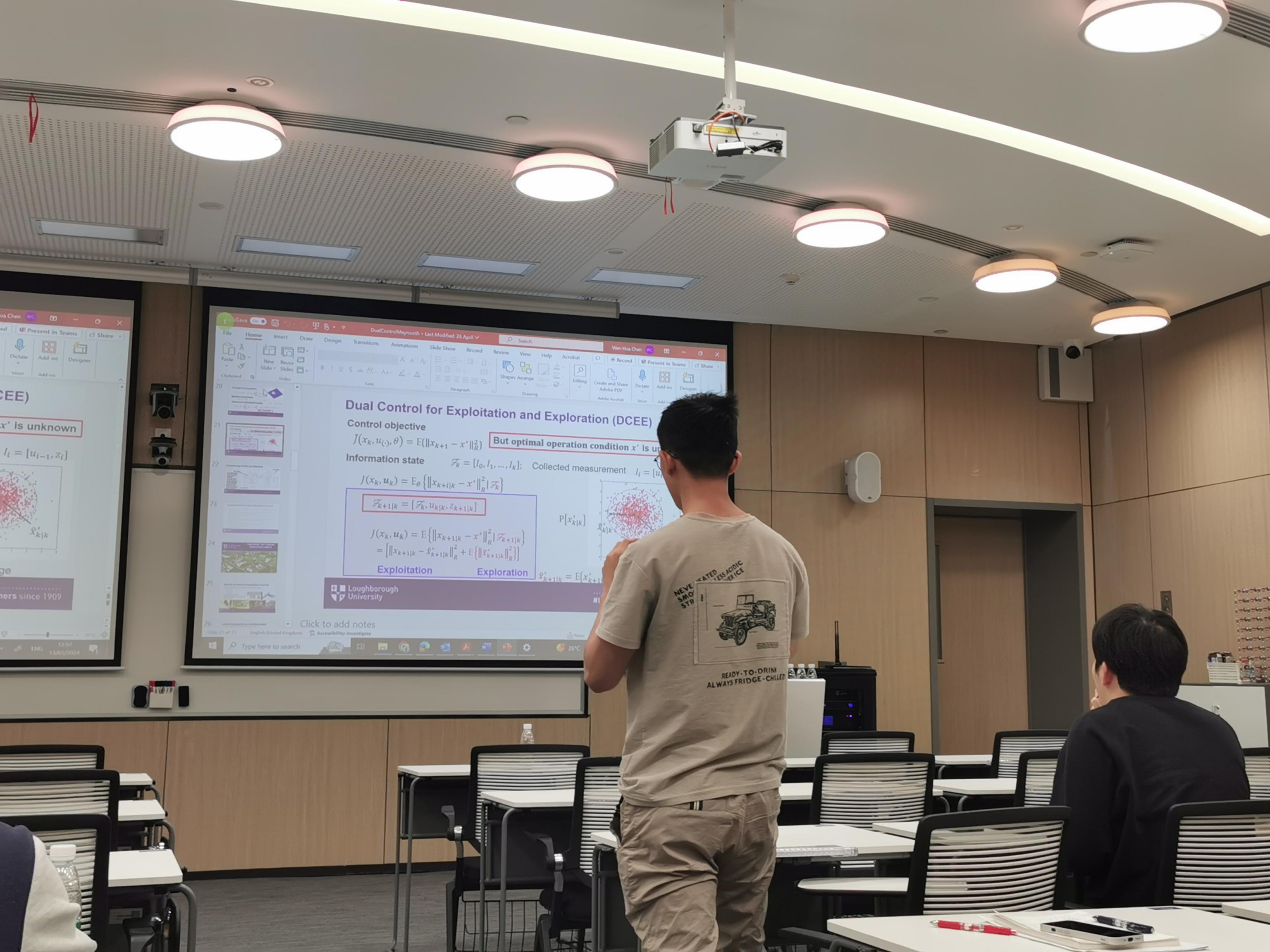
图3 陈教授与现场师生的问答互动环节
在演讲结束后,陈教授还针对学生们提出的问题进行了深入的讲解,为同学们提供了新的研究思路。我们由衷感谢陈教授的到访,并期待未来有更多的学术交流与合作机会。
讲座信息如下
时间:2024年5月13日(周一)上午10:40am-12:00pm
Time: 10:40am-12:00pm, Monday, May 13, 2024
地点:云谷校区E10-205
Venue: E10-205, Yungu Campus
主持人: 工学院 赵世钰 博士
Host: Dr. Shiyu Zhao, School of Engineering
语言:英文
Language: English
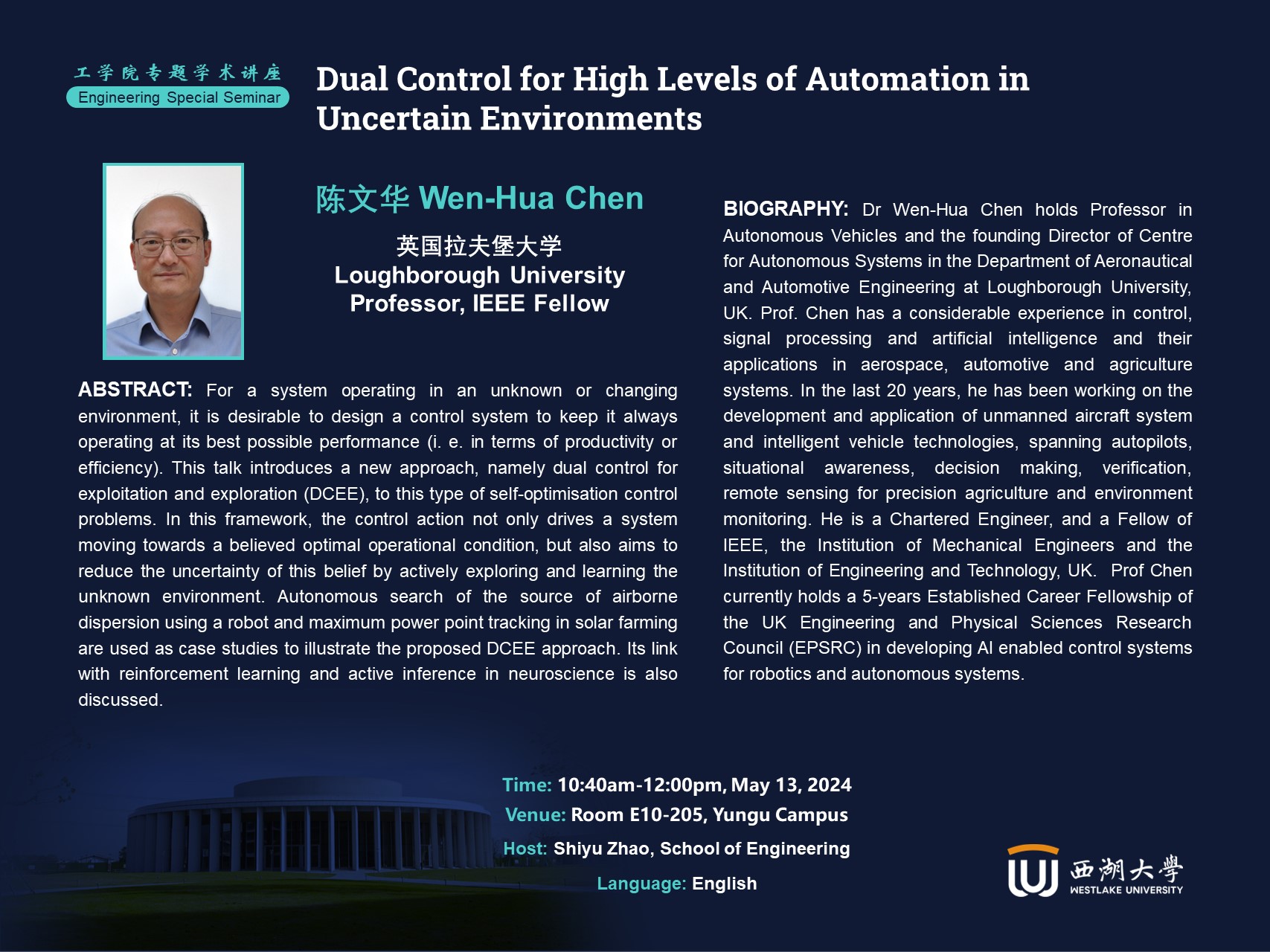
主讲人简介/Biography:
Dr Wen-Hua Chen holds Professor in Autonomous Vehicles and the founding Director of Centre for Autonomous Systems in the Department of Aeronautical and Automotive Engineering at Loughborough University, UK. Prof. Chen has a considerable experience in control, signal processing and artificial intelligence and their applications in aerospace, automotive and agriculture systems. In the last 20 years, he has been working on the development and application of unmanned aircraft system and intelligent vehicle technologies, spanning autopilots, situational awareness, decision making, verification, remote sensing for precision agriculture and environment monitoring. He is a Chartered Engineer, and a Fellow of IEEE, the Institution of Mechanical Engineers and the Institution of Engineering and Technology, UK. Prof Chen currently holds a 5-years Established Career Fellowship of the UK Engineering and Physical Sciences Research Council (EPSRC) in developing AI enabled control systems for robotics and autonomous systems.
讲座摘要/Abstract:
For a system operating in an unknown or changing environment, it is desirable to design a control system to keep it always operating at its best possible performance (i. e. in terms of productivity or efficiency). This talk introduces a new approach, namely dual control for exploitation and exploration (DCEE), to this type of self-optimisation control problems. In this framework, the control action not only drives a system moving towards a believed optimal operational condition, but also aims to reduce the uncertainty of this belief by actively exploring and learning the unknown environment. Autonomous search of the source of airborne dispersion using a robot and maximum power point tracking in solar farming are used as case studies to illustrate the proposed DCEE approach. Its link with reinforcement learning and active inference in neuroscience is also discussed.
西湖大学官网讲座信息链接:Dual Control for High Levels of Automation in Uncertain Environments